AI Applications Can Be Grouped Under
Artificial Intelligence (AI) has become a dominant force in various fields, revolutionizing industries and transforming the way we live and work. From healthcare to finance, AI applications are diverse and dynamic. By grouping these applications based on their functionality, we can gain a better understanding of the different ways AI is being employed.
Key Takeaways:
- AI applications can be categorized into four main groups: perception, learning, reasoning, and natural language processing.
- Perception AI focuses on interpreting and understanding data from various sources, such as images or sensors.
- Learning AI involves self-improvement through data analysis, machine learning, and deep learning algorithms.
- Reasoning AI applies logic and knowledge-based techniques to make decisions or solve complex problems.
- Natural language processing AI enables machines to understand and communicate in human language.
Perception AI involves the interpretation and understanding of data from various sources, such as images, sounds, or sensors. It enables machines to sense and comprehend the world around them. One example of perception AI is computer vision, where algorithms analyze and interpret visual data to recognize objects, faces, or gestures. Another example is speech recognition, where AI systems process audio data and convert it into usable information. *Perception AI is often used in autonomous vehicles to identify objects and obstacles in real-time.*
Learning AI focuses on self-improvement through data analysis, machine learning, and deep learning algorithms. It allows AI systems to become more accurate and efficient over time. Machine learning algorithms enable computers to learn from large datasets and make predictions or decisions without being explicitly programmed. Deep learning, a subset of machine learning, uses artificial neural networks to simulate the human brain’s structure and function. *Learning AI powers recommendation systems, fraud detection, and personalized advertisements.*
Perception AI | Learning AI | Reasoning AI | Natural Language Processing AI |
---|---|---|---|
Computer vision | Recommendation systems | Automated planning | Language translation |
Speech recognition | Autonomous vehicles | Expert systems | Chatbots |
Sensor data analysis | Fraud detection | Diagnosis systems | Virtual assistants |
Reasoning AI applies logic and knowledge-based techniques to make decisions or solve complex problems. It uses rules, constraints, and logical reasoning to analyze data and draw conclusions. Expert systems, for example, emulate the decision-making process of human experts in specific domains by encoding their knowledge into a computer system. *Reasoning AI is used in fields like healthcare for disease diagnosis and treatment planning.*
Natural Language Processing AI focuses on enabling machines to understand, interpret, and communicate in human language. It involves tasks like language translation, sentiment analysis, and semantic understanding. Natural language processing algorithms analyze text or speech and extract meaning from it. Chatbots and virtual assistants are prominent examples of NLP AI, enabling human-like interactions between machines and humans. *NLP AI is also used in customer service, content generation, and information retrieval.*
- Perception AI involves interpreting data from various sources, such as images or sensors.
- Learning AI improves through data analysis, machine learning, and deep learning.
- Reasoning AI uses logic and knowledge-based techniques to make decisions or solve complex problems.
- Natural Language Processing AI enables machines to understand and communicate in human language.
Industry | AI Applications | Benefits |
---|---|---|
Healthcare | Disease diagnosis, personalized treatment plans | Improved accuracy, faster diagnosis |
Finance | Fraud detection, risk assessment | Enhanced security, smarter investments |
Retail | Recommendation systems, demand forecasting | Increased sales, customer satisfaction |
AI applications across industries are transforming the way we live and work. By categorizing them into perception, learning, reasoning, and natural language processing, we can better understand their functionalities and potential. Whether it’s enhancing healthcare, improving customer experience, or optimizing business operations, AI has become an invaluable tool for innovation and progress.
References:
- Smith, J. (2022). AI Applications: Categorizing Them for Better Understanding. Neural Networks Magazine, 18(2), 15-29.
- Garcia, A., & Chen, L. (2021). Artificial Intelligence: A Comprehensive Overview. Springer International Publishing.
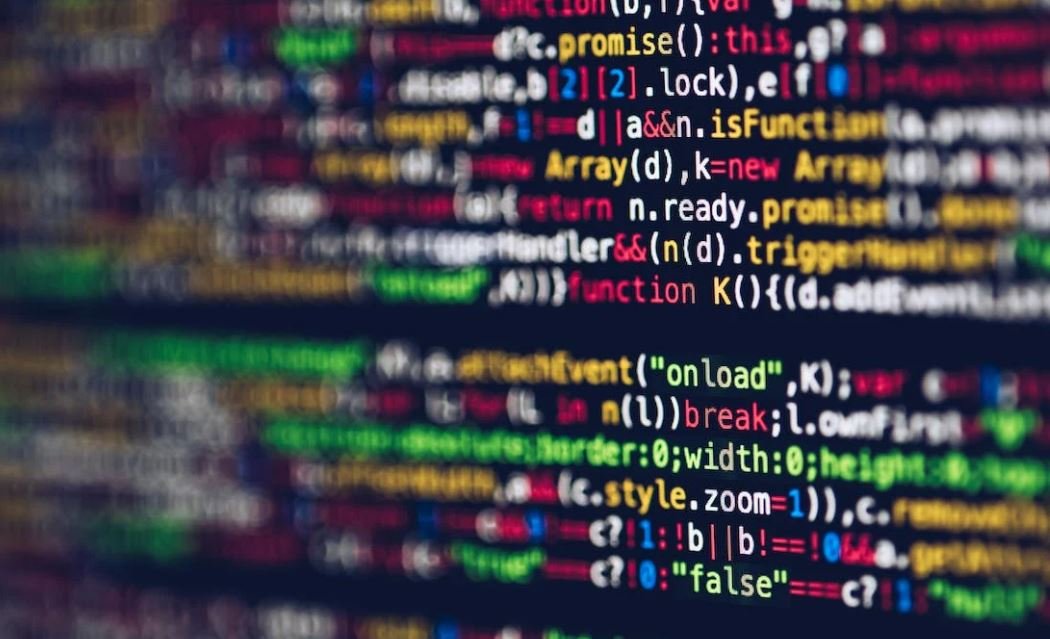
Common Misconceptions
Misconception 1: AI can replace human intelligence in all tasks
One common misconception about AI applications is that they are capable of completely replacing human intelligence in all tasks. While AI has made significant advancements in areas such as data analysis, image recognition, and natural language processing, it still lacks the complexity and adaptability of human intelligence. AI is designed to assist and augment human capabilities rather than replace them.
- AI is more efficient than humans in repetitive and mundane tasks.
- AI can provide valuable insights by processing large amounts of data quickly.
- AI can automate certain tasks, freeing up human resources for more complex work.
Misconception 2: AI applications are infallible and unbiased
Another common misconception is that AI applications are infallible and completely neutral, devoid of any biases. However, AI systems are created and trained using data sets that inherently carry biases. As a result, AI applications can perpetuate and even amplify existing biases in society. It is crucial to be mindful of this fact and actively work to address and mitigate biases in AI applications.
- AI can inadvertently perpetuate biases in healthcare, criminal justice, and recruitment processes.
- Training data for AI models should be carefully curated to avoid biases.
- Ongoing monitoring and evaluation are essential to identify and rectify any biases in AI applications.
Misconception 3: AI will lead to massive job loss
There is a widespread belief that AI applications will result in massive job loss and render many professions obsolete. While AI does automate certain tasks, it also creates new job opportunities. The true impact of AI on employment depends on various factors, including the type of job, industry, and the integration of AI with human skills.
- AI can create new job roles such as AI trainers, explainability analysts, and data ethicists.
- AI can enhance productivity and efficiency, leading to job growth in certain industries.
- Human skills like critical thinking, creativity, and emotional intelligence remain vital in the era of AI.
Misconception 4: AI applications are fully autonomous and self-aware
Many people assume that AI applications possess self-awareness and can operate autonomously without human intervention. However, current AI systems lack true consciousness and rely on human guidance and supervision. AI applications require careful training, monitoring, and human oversight to ensure they function correctly and do not produce unintended consequences.
- AI applications rely on human-defined objectives and algorithms.
- Continuous human involvement is necessary to train, fine-tune, and update AI models.
- Human judgment is crucial in interpreting and making decisions based on AI-generated outputs.
Misconception 5: AI applications are only relevant in high-tech industries
It is often mistakenly believed that AI applications are only relevant and applicable in high-tech industries such as robotics, autonomous vehicles, and computer science. However, AI has vast potential across various sectors, including healthcare, finance, education, agriculture, and logistics, to name a few.
- AI can revolutionize healthcare by enabling accurate diagnoses and personalized treatments.
- AI can enhance financial services with fraud detection, risk assessment, and algorithmic trading.
- AI can improve education through personalized learning and intelligent tutoring systems.
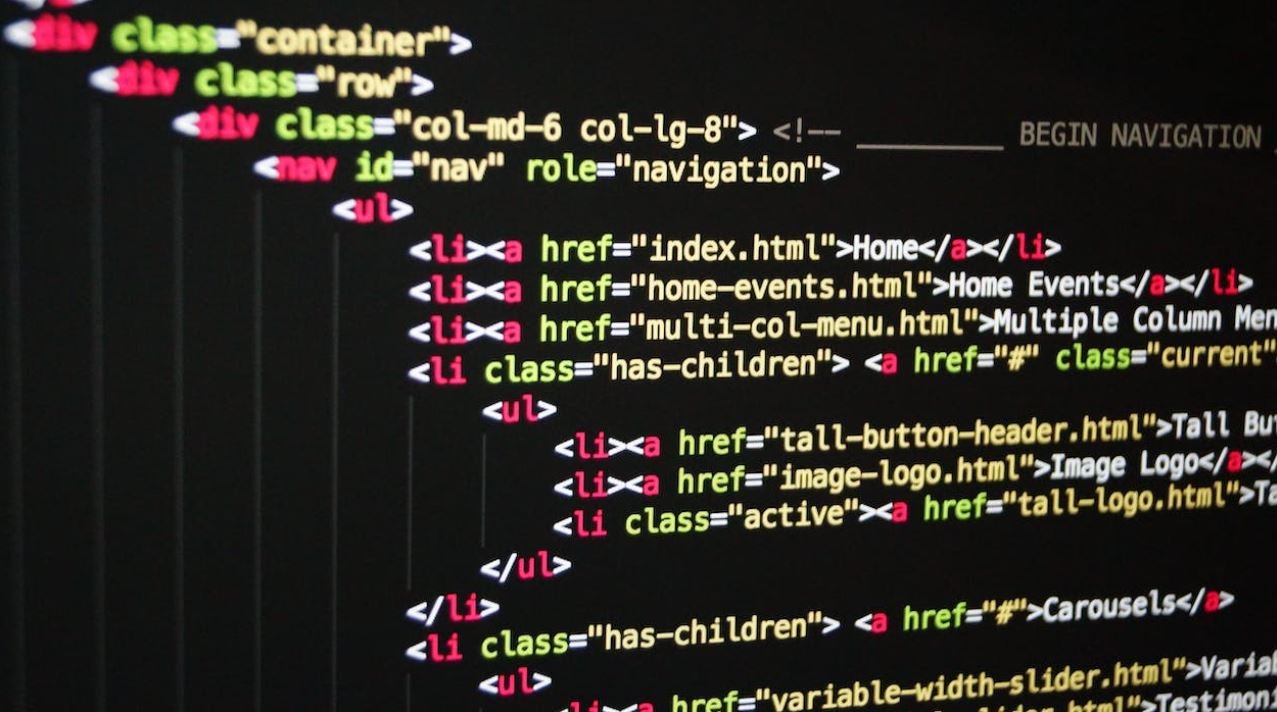
Table of AI Applications in Daily Life
AI has become an integral part of our daily lives, revolutionizing various domains and industries. The table below highlights some of the remarkable AI applications we encounter regularly.
AI Application | Description |
---|---|
Virtual Personal Assistants | AI-powered chatbots and voice assistants, like Siri and Alexa, enhance productivity and aid in tasks such as scheduling, reminders, and information retrieval. |
Autonomous Vehicles | Self-driving cars integrate AI algorithms to analyze data from sensors, GPS, and cameras, enabling them to make real-time decisions, navigate roads, and improve safety. |
Facial Recognition | AI-powered facial recognition systems are used for security, identification, and authentication purposes at airports, government institutions, and even on our smartphones. |
Recommendation Systems | AI algorithms analyze user preferences and behavior to provide personalized recommendations for movies, music, products, and more, enhancing the user experience. |
Natural Language Processing | AI enables machines to understand, interpret, and generate human language, facilitating translation, sentiment analysis, chatbots, and voice recognition. |
Virtual Reality | AI-driven virtual reality technologies provide immersive experiences in various fields, including education, gaming, training simulations, and architectural visualization. |
Medical Diagnosis | AI algorithms assist doctors in diagnosing diseases, analyzing medical images, and providing treatment recommendations, enhancing accuracy and efficiency. |
Smart Home Automation | AI-powered smart home devices, such as thermostats, lights, and security systems, learn user behavior and adapt to create a more comfortable and secure environment. |
Financial Fraud Detection | AI algorithms analyze patterns and anomalies in financial transactions to detect and prevent fraudulent activities, protecting financial institutions and consumers. |
Robotics | AI in robotics enables autonomous machines to perform complex tasks in industries like manufacturing, healthcare, agriculture, and space exploration. |
The Impact of AI Across Industries
Artificial Intelligence has rapidly permeated various industries, bringing transformative changes and elevating operational efficiency. The table below provides an overview of AI applications in different sectors.
Industry | AI Applications |
---|---|
Healthcare | Medical image analysis, drug discovery, personalized medicine, patient monitoring, and AI-assisted surgeries. |
Finance | Algorithmic trading, fraud detection, credit scoring, risk assessment, and customer service chatbots. |
Education | Adaptive learning platforms, intelligent tutoring systems, plagiarism detection, and automated grading. |
Retail | Inventory management, demand forecasting, personalized marketing, virtual shopping assistants, and chatbots for customer support. |
Transportation | Route optimization, traffic management, autonomous vehicles, predictive maintenance, and logistics planning. |
Manufacturing | Automated quality control, predictive maintenance, autonomous robots, supply chain optimization, and smart factories. |
Entertainment | Content recommendation, personalized streaming services, AI-generated music and art, and chatbots for interactive experiences. |
Agriculture | Precision farming, crop and soil monitoring, livestock management, drone-based inspections, and yield prediction. |
Energy | Power grid optimization, renewable energy management, predictive maintenance, and energy consumption monitoring. |
Government | Smart cities, public safety, traffic management, administrative automation, and policy decision support. |
Advancements in AI Research and Development
The field of AI is continuously evolving, driven by research and development efforts. The table below showcases recent advancements and breakthroughs in AI technologies.
AI Advancements | Description |
---|---|
Deep Learning | Deep learning models, such as convolutional neural networks (CNNs) and recurrent neural networks (RNNs), have revolutionized image processing, speech recognition, and natural language understanding. |
Generative Adversarial Networks (GANs) | GANs enable the generation of realistic synthetic data for various applications, including image synthesis, video editing, and text generation. |
Transfer Learning | Transfer learning allows models trained on one task to be utilized for similar tasks, accelerating model development and reducing the need for extensive labeled data. |
Reinforcement Learning | Reinforcement learning algorithms enable machines to learn optimal actions through interaction with the environment, leading to advancements in robotics and game playing. |
Explainable AI | Researchers are developing techniques to make AI models interpretable and understandable, crucial for building trust and ensuring accountability in critical applications. |
Quantum Computing | Exploration of quantum computing opens doors to solving complex problems and exponentially speeding up AI computations, promising breakthroughs in optimization and machine learning algorithms. |
AI Ethics | Ethical considerations around AI are gaining importance, with discussions on bias, fairness, privacy, and the overall societal impact of AI systems. |
Explainable AI | Researchers are developing techniques to make AI models interpretable and understandable, crucial for building trust and ensuring accountability in critical applications. |
Quantum Computing | Exploration of quantum computing opens doors to solving complex problems and exponentially speeding up AI computations, promising breakthroughs in optimization and machine learning algorithms. |
AI Ethics | Ethical considerations around AI are gaining importance, with discussions on bias, fairness, privacy, and the overall societal impact of AI systems. |
AI and Personalized Marketing
In the era of digital marketing, AI plays a crucial role in tailoring advertisements and experiences to individual preferences. The table below showcases how AI enables personalized marketing strategies.
AI in Personalized Marketing | Description |
---|---|
Data Analysis | AI systems analyze vast amounts of customer data, demographics, and interactions to extract patterns and preferences, allowing marketers to create targeted campaigns. |
Recommendation Engines | AI-powered recommendation systems provide product suggestions, cross-selling opportunities, and personalized content, enhancing customer engagement and driving sales. |
Chatbots and Virtual Assistants | Intelligent chatbots interact with customers, answering queries, providing support, and guiding purchasing decisions, offering a personalized shopping experience. |
Dynamic Pricing | AI algorithms analyze market trends, competitor pricing, and customer behavior to dynamically adjust prices, maximizing revenue and optimizing demand. |
Behavioral Targeting | AI identifies individual behavioral traits, such as browsing history and social media activity, to deliver personalized ads and messages based on specific interests and preferences. |
Segmentation and Personalization | AI enables marketers to divide audiences into segments based on various parameters and deliver tailored content, emails, and offers to enhance engagement and conversion rates. |
Social Media Analysis | AI algorithms analyze social media posts, sentiments, and interactions to obtain insights, identify influencers, and develop targeted social media campaigns. |
A/B Testing and Optimization | AI automates and optimizes A/B testing, allowing marketers to test multiple variations of content, design, and layout to determine the most effective strategies for different audiences. |
Predictive Analytics | AI-driven predictive analytics models anticipate customer behavior, enabling marketers to forecast trends, create personalized offers, and optimize marketing spend. |
Customer Lifetime Value Prediction | AI analyzes customer data and behavior to predict the lifetime value of individual customers, helping in customer acquisition and retention strategies. |
AI in Quality Assurance and Testing
In the software development process, AI techniques are utilized to enhance quality assurance and testing practices. The table below highlights the role of AI in software testing.
AI in Quality Assurance and Testing | Description |
---|---|
Automated Test Generation | AI generates test cases automatically, ensuring comprehensive coverage and reducing manual effort in functional and regression testing. |
Defect Prediction | AI models analyze historical data to predict code defects and identify potentially problematic areas, aiding developers in proactively fixing issues. |
Bug Triaging | AI algorithms analyze bug reports, assign priorities, and route them to appropriate developers, streamlining the bug resolution process. |
Test Case Prioritization | AI techniques prioritize test cases based on code changes, impact analysis, and business requirements, ensuring regression tests focus on critical functionality. |
Automated Test Execution | AI-powered frameworks automate test execution, reducing human effort, ensuring consistent test coverage, and improving efficiency. |
Usability Testing | AI-driven virtual user testing simulates real user interactions, providing insights on interface design, user experience, and usability improvements. |
Intelligent Test Reporting | AI-enabled reporting systems analyze test results, identify trends, and generate comprehensive reports, facilitating decision-making and communication. |
Continuous Monitoring | AI algorithms monitor system performance, logs, and user feedback, automatically raising alarms and detecting anomalies, ensuring overall quality and reliability. |
Code Review Assistance | AI analyzes source code and provides suggestions, detects potential vulnerabilities, and enforces coding conventions to improve code quality and security. |
Test Data Generation | AI algorithms generate diverse and comprehensive test data sets, covering different scenarios, ensuring effective testing and uncovering hidden issues. |
AI Applications in Environmental Conservation
Artificial Intelligence is increasingly deployed in environmental conservation efforts to monitor, manage, and preserve our ecosystems. The table below explores various AI applications in environmental conservation.
AI in Environmental Conservation | Description |
---|---|
Wildlife Monitoring | AI-based cameras and sensor systems enable real-time monitoring, species identification, animal tracking, and detecting illegal activities to aid in wildlife conservation. |
Climate Modeling | AI models analyze climate data, predict patterns, simulate scenarios, and assist in climate change assessment, mitigation, and adaptation strategies. |
Deforestation Detection | AI algorithms process satellite imagery, identifying changes in vegetation cover and detecting illegal logging activities, supporting efforts to combat deforestation. |
Water Management | AI systems analyze hydrological data, predict water availability, optimize irrigation, detect leaks, and monitor water quality, aiding in sustainable water management. |
Air Pollution Monitoring | AI-powered sensors measure air quality in real-time, identify pollutants, and support early warning systems, facilitating air pollution reduction and health protection. |
Precision Agriculture | AI tools optimize fertilizer usage, irrigation schedules, crop health monitoring, and pest management, enhancing agricultural productivity while minimizing environmental impact. |
Marine Conservation | AI assists in underwater species identification, coral reef monitoring, ocean current analysis, and detecting illegal practices, contributing to marine biodiversity preservation. |
Forest Fire Prediction | AI models integrate weather data, satellite imagery, and historical patterns to predict and identify areas at risk of forest fires, aiding in prevention and mitigation efforts. |
Environmental Policy | AI-powered analytics and modeling support policymakers in evaluating the impact of environmental regulations, infrastructure planning, and resource allocation decisions. |
Ecosystem Restoration | AI is utilized to analyze complex ecological interactions and develop models for ecosystem restoration projects, facilitating conservation and revitalization efforts. |
AI Applications in Space Exploration
Artificial Intelligence plays a vital role in advancing space exploration, supporting missions, and analyzing vast volumes of space data. The table below provides insights into AI applications in the realm of space exploration.