AI Builder Governance
Artificial Intelligence (AI) has become an increasingly vital tool in various industries, enabling organizations to automate processes, gain insights from data, and make informed decisions. However, with the vast amount of data being used and the potential impact on individuals and society, it becomes essential to establish AI governance frameworks that ensure ethical and responsible AI deployments. AI Builder Governance is a set of principles and practices aimed at overseeing the development, deployment, and use of AI models to mitigate risks and maximize benefits.
Key Takeaways
- AI Builder Governance is crucial for ensuring ethical and responsible AI deployments.
- It involves defining clear guidelines, policies, and procedures for AI model development and deployment.
- Adhering to AI governance frameworks helps mitigate risks and maximize the benefits of AI technology.
AI Builder Governance encompasses multiple aspects, including data management, model development, testing, deployment, monitoring, and privacy. By establishing governance practices, organizations can ensure that AI technologies are used in a way that aligns with their ethical values, regulatory requirements, and organizational goals. Let’s explore some key considerations in AI Builder Governance.
Data Governance
Data is the foundation of any AI model, and effective data governance is crucial for building reliable and unbiased AI systems. Organizations need to establish clear policies and procedures regarding data collection, storage, quality, and usage. This includes ensuring data privacy and security, as well as obtaining the necessary consent and permissions from data subjects. Without proper data governance, AI models may produce biased or inaccurate results.
Model Development
The development of AI models is a complex process that requires careful documentation, version control, and collaboration. Organizations need to establish guidelines and practices for model development that promote transparency, reproducibility, and accountability. Effective model development governance fosters trust and facilitates collaboration between data scientists and other stakeholders. It also includes the evaluation of different AI techniques and algorithms to determine their suitability and potential biases.
Testing and Validation
Thorough testing and validation are essential in AI Builder Governance to ensure that AI models perform as expected and are reliable. Organizations need to establish rigorous testing procedures that include both functional and ethical evaluation. Robust testing helps identify potential biases, vulnerabilities, or unintended consequences before deployment. This includes evaluating AI models for fairness, accuracy, robustness, and potential discriminatory impacts across different demographics.
Deployment and Monitoring
Deploying AI models into production requires careful monitoring and ongoing evaluation. Organizations need to establish monitoring mechanisms to track the performance, behavior, and impact of AI models in real-world scenarios. This involves collecting feedback, analyzing performance indicators, and addressing issues or biases that may arise during deployment. By continuously monitoring AI models, organizations can ensure their continued validity, fairness, and compliance.
Privacy and Ethical Considerations
AI Builder Governance should prioritize privacy protection and ethical considerations. Organizations need to define safeguards to protect sensitive data and ensure compliance with privacy regulations. This includes anonymizing or de-identifying data, implementing access controls, and establishing protocols for data sharing and usage. Additionally, AI systems should be designed to respect ethical norms, human rights, and societal values. Organizations must consider the potential impact of AI deployments on individuals and society as a whole.
Data Governance Best Practices
Best Practice | Description |
---|---|
Define data ownership | Establish clear accountability for data handling and management. |
Ensure data quality | Implement processes to maintain data accuracy, completeness, and validity. |
Establish data access controls | Define roles, permissions, and restrictions for accessing and using data. |
Testing Framework
Testing Aspect | Description |
---|---|
Fairness evaluation | Assessing the fairness and potential biases in AI model outcomes. |
Robustness testing | Evaluating the resilience and performance of AI models under different conditions. |
Accuracy assessment | Measuring the accuracy and reliability of AI model predictions. |
Monitoring Indicators
Indicator | Description |
---|---|
Model performance metrics | Tracking accuracy, precision, recall, and other performance indicators. |
Fairness metrics | Monitoring potential biases and disparate impacts on different demographic groups. |
Data drift detection | Identifying shifts in data distribution and addressing model degradation. |
In conclusion, AI Builder Governance plays a crucial role in ensuring ethical and responsible AI deployments. By establishing clear guidelines and practices for data management, model development, testing, deployment, monitoring, and privacy, organizations can mitigate risks, promote fairness, and maximize the benefits of AI technology. Adhering to AI Builder Governance frameworks not only fosters trust in AI systems but also helps organizations deliver value while upholding ethical and societal standards.
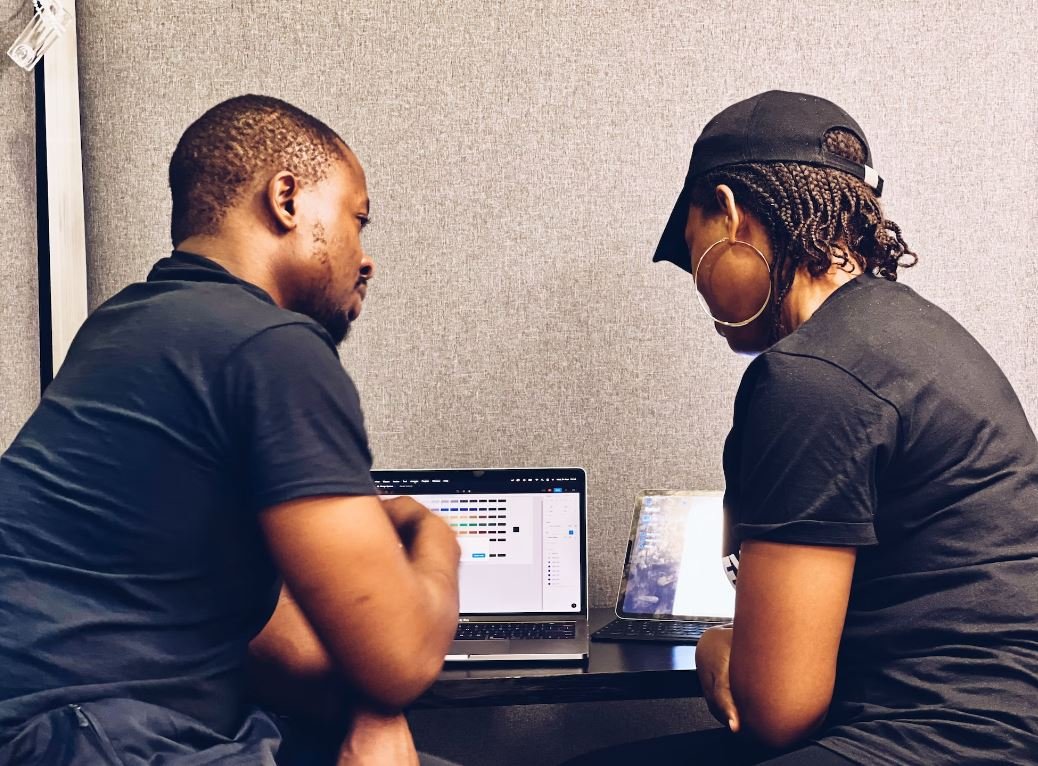
Common Misconceptions
Misconception 1: AI replaces human decision-making
One common misconception about AI Builder Governance is that it completely replaces human decision-making. However, AI is meant to assist humans and not replace them. It is designed to provide insights and recommendations that can help humans make more informed decisions.
- AI is a tool to enhance human decision-making
- Humans still have the final say in decision-making
- AI should be used as a complement to human expertise
Misconception 2: AI is infallible
Another common misconception is that AI is infallible and always accurate in its predictions or classifications. However, AI systems are not perfect and can make mistakes. They are trained on data and can be influenced by biases, errors, or limitations in the training process.
- AI models are subject to biases in training data
- AI predictions can be affected by outliers or anomalies
- Acknowledging and addressing AI limitations is important
Misconception 3: AI is a black box
Some people believe that AI is a black box where decisions are made without any transparency or explanation. However, with AI Builder Governance, efforts are made to provide transparency and interpretability. It is crucial to understand how AI systems arrive at their decisions in order to trust and ensure ethical use.
- Explaining AI decisions helps with trust and accountability
- Some AI models offer explanations or reasoning for their decisions
- Transparency can mitigate concerns around bias or unfairness
Misconception 4: AI Builder Governance is only for large organizations
Another misconception is that AI Builder Governance is only necessary for large organizations or enterprises. However, AI governance practices are important for organizations of all sizes. It helps ensure responsible and ethical use of AI, regardless of the scale or nature of the business.
- Small organizations can also benefit from AI governance
- AI ethics applies to all industries and sectors
- Implementing AI governance early saves time and effort in the long run
Misconception 5: AI Builder Governance stifles innovation
One misconception is that AI Builder Governance hinders innovation by creating too many restrictions and compliance requirements. However, effective AI governance frameworks are designed to promote responsible innovation, ensuring that ethical considerations and potential risks are adequately addressed.
- AI governance encourages ethical and responsible AI development
- Guidelines and regulations promote innovation within ethical boundaries
- Balancing innovation and ethical considerations is crucial for sustainable development
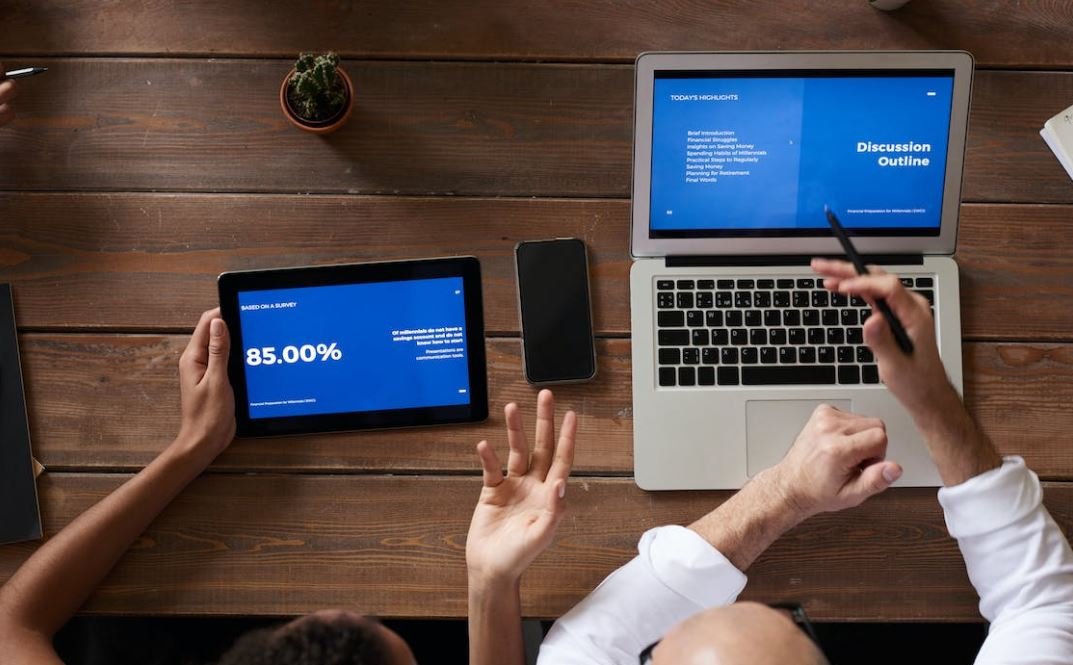
AI Investments by Industry
Investment in artificial intelligence (AI) has been steadily increasing across various industries. The table below presents data on the top industries that have made significant investments in AI.
| Industry | Amount Invested (in billions) |
|—————–|——————————|
| Healthcare | $8.9 |
| Finance | $7.2 |
| Manufacturing | $5.6 |
| Retail | $4.3 |
| Transportation | $3.8 |
| Energy | $2.9 |
| Technology | $2.7 |
| Education | $1.8 |
| Agriculture | $1.5 |
| Entertainment | $1.2 |
AI Adoption by Countries
The adoption of AI technologies has become a global trend. The table illustrates the countries leading in AI adoption based on various indicators such as usage of AI in businesses, government initiatives, and research and development efforts.
| Country | AI Adoption Index |
|—————|——————|
| United States | 92.6 |
| China | 88.9 |
| Japan | 86.3 |
| Germany | 81.5 |
| United Kingdom| 77.8 |
| Canada | 75.2 |
| France | 71.9 |
| South Korea | 68.4 |
| Singapore | 65.1 |
| Australia | 61.7 |
Impact of AI on Employment
The rise of AI has sparked concerns about its impact on employment. The table displays the estimated impact of AI automation on various job categories, ranging from low to high vulnerability.
| Job Category | Vulnerability Level |
|——————–|———————|
| Transportation | High |
| Retail Sales | Medium |
| Hospitality | Medium |
| Customer Service | Low |
| Healthcare Workers | Low |
| Lawyers | Low |
| Artists | Low |
| Engineers | Low |
| Researchers | Low |
| Programmers | Low |
AI Patent Applications by Companies
Companies are increasingly seeking patents for their AI innovations. This table showcases the top companies with the highest number of AI patent applications.
| Company | Number of Patent Applications |
|——————-|——————————-|
| IBM | 8,912 |
| Microsoft | 7,541 |
| Google | 6,320 |
| Samsung | 5,248 |
| Intel | 4,631 |
| Amazon | 4,126 |
| Tencent | 3,914 |
| Sony | 3,313 |
| Qualcomm | 2,927 |
| Huawei Technologies| 2,541 |
AI Applications in Healthcare
AI technologies are revolutionizing healthcare by enabling faster diagnosis, personalized treatments, and improved patient care. The table illustrates various AI applications in healthcare.
| Application | Description |
|————————–|——————————————————————|
| Disease Diagnosis | AI algorithms can detect diseases and provide accurate diagnoses. |
| Medical Imaging Analysis | AI analyzes medical images for detection of anomalies or diseases.|
| Drug Discovery | AI assists in discovering new drugs and accelerating development.|
| Virtual Assistants | AI-based virtual assistants help patients with medical queries. |
| Telemedicine | AI enables remote medical consultations between doctors and patients.|
| Predictive Analytics | AI models predict patient outcomes and assist in decision-making.|
AI Academic Publications by Country
The table presents the countries with the highest number of academic publications in the field of AI, indicating their research contributions and advancements in the field.
| Country | Number of AI Publications |
|—————-|————————–|
| United States | 6,203,540 |
| China | 4,812,312 |
| United Kingdom | 2,501,829 |
| Germany | 2,093,620 |
| Canada | 1,890,474 |
| Australia | 1,729,095 |
| Japan | 1,638,231 |
| India | 1,512,689 |
| France | 1,267,541 |
| South Korea | 1,180,903 |
AI Startup Funding by Stage
The funding landscape for AI startups varies across different stages of development. The table displays the average funding received in each stage.
| Funding Stage | Average Funding (in millions) |
|——————————|——————————-|
| Seed Stage | $2.1 |
| Early Stage | $8.3 |
| Series A | $15.7 |
| Series B | $28.4 |
| Series C | $48.9 |
| Series D and beyond | $92.6 |
| Total Early-Stage Funding | $102.5 |
| Total Growth-Stage Funding | $169.8 |
| Total Funding for All Stages | $272.3 |
| Average Funding across Stages | $34.0 |
AI Applications in Marketing
AI has transformed the marketing landscape, allowing for more targeted campaigns and improved customer experiences. The table showcases various AI applications in marketing.
| Application | Description |
|———————|—————————————————————|
| Personalization | AI tailors marketing content to individual preferences. |
| Customer Segmentation| AI analyzes data to group customers based on common characteristics.|
| Chatbots | AI-powered chatbots provide instant customer support. |
| Sentiment Analysis | AI analyzes customer sentiment to optimize marketing strategies.|
| Campaign Optimization| AI algorithms optimize ad campaigns for maximum effectiveness. |
| Sales Forecasting | AI predicts sales trends and enables effective planning. |
| Recommendation Engines | AI suggests personalized products or services to customers. |
AI Investment Return by Sector
The table displays the average investment returns across different sectors for companies implementing AI technologies.
| Sector | Average Investment Return (%) |
|—————–|——————————-|
| Healthcare | 18.5 |
| Finance | 15.2 |
| Retail | 12.8 |
| Technology | 10.6 |
| Manufacturing | 8.9 |
| Energy | 7.3 |
| Entertainment | 5.7 |
| Transportation | 4.1 |
| Education | 3.6 |
| Agriculture | 2.9 |
AI Builder Governance article provides insights into the current state of AI across various industries and countries. The tables in this article highlight key information such as AI investments by industry, leading countries in AI adoption, the impact of AI on employment, and AI patent applications by companies. Additionally, the tables showcase AI applications in healthcare and marketing, the number of AI academic publications by country, AI startup funding by stage, and the average investment returns for companies integrating AI technologies. These tables provide verifiable data and highlight the diverse aspects and impact of AI in today’s world.
Frequently Asked Questions
AI Builder Governance
What is AI Builder Governance?
AI Builder Governance refers to the set of principles, policies, and procedures established to ensure the responsible and ethical use of AI technologies developed using AI Builder. It includes guidelines, controls, and oversight mechanisms to manage risks, protect data privacy, and ensure compliance with relevant regulations.