AI Enterprise Workflow
The integration of artificial intelligence (AI) into enterprise workflows has revolutionized the way businesses operate. By leveraging advanced algorithms and machine learning techniques, organizations can automate repetitive tasks, extract valuable insights from data, and improve decision-making processes. This article provides an overview of AI enterprise workflow and highlights its key benefits and challenges.
Key Takeaways:
- AI integration enhances efficiency and productivity within enterprise workflows.
- Automated data analysis can uncover valuable insights and patterns.
- Skilled data scientists and AI experts are crucial for successful implementation.
- Privacy and ethical considerations must be addressed when using AI.
In today’s fast-paced business environment, organizations are constantly seeking new ways to streamline their processes and stay ahead of the competition. AI enterprise workflow offers a solution by leveraging technology to automate and optimize various aspects of business operations. From data analysis to customer service, AI can be integrated into any workflow to enhance efficiency and productivity.
AI technologies, such as machine learning and natural language processing, enable computers to analyze large datasets and make intelligent predictions. This automated data analysis not only saves time but also uncovers valuable insights and patterns that may have gone unnoticed. By leveraging AI algorithms, businesses can make data-driven decisions and gain a competitive advantage in their respective industries.
*AI can also assist in data cleaning and preprocessing to ensure the accuracy and quality of the input data.*
Implementation Challenges:
- Availability of skilled data scientists and AI experts for successful implementation.
- Addressing privacy concerns and ensuring data security.
- Building and maintaining robust AI infrastructure.
Implementing AI enterprise workflow successfully requires the expertise of skilled data scientists and AI specialists who can develop and deploy sophisticated algorithms tailored to an organization’s specific needs. These professionals possess the knowledge and experience to extract meaningful insights from data, manage AI models, and optimize their performance.
*The rapid pace of technological advancements poses challenges for organizations to keep up with skill requirements.*
Privacy and ethical considerations are crucial aspects to be addressed when integrating AI into enterprise workflows. Organizations must ensure that data privacy laws and regulations are followed, and proper security measures are in place to protect sensitive information. Additionally, ethical concerns related to bias, fairness, and transparency require careful consideration to mitigate potential risks associated with AI implementation.
Building a robust AI infrastructure is another challenge organizations face during implementation. This includes acquiring the necessary hardware, software, and computing resources to support complex AI algorithms and models. Organizations must also maintain an infrastructure that can scale to handle increasing data volumes and evolving AI technologies.
*Efficient resource allocation is vital to ensure the smooth functioning of an AI-powered enterprise workflow.*
Benefits of AI Enterprise Workflow | Challenges of AI Enterprise Workflow |
---|---|
|
|
In conclusion, AI enterprise workflow offers immense potential for organizations to automate and optimize their operations. By integrating AI technologies into their workflows, businesses can achieve greater efficiency, uncover valuable insights, and improve decision-making processes. However, successful implementation requires the expertise of skilled professionals, addressing privacy and ethical concerns, and building robust infrastructure.
*Embracing AI in enterprise workflows is no longer a luxury but a necessity in the digital era, enabling organizations to thrive and stay ahead of the competition.*
Impact of AI on Various Industries |
---|
|
By embracing AI in various industries, organizations can unlock new opportunities, drive innovation, and improve customer satisfaction. The future holds immense potential for AI integration, and businesses that leverage its power in their enterprise workflows will undoubtedly gain a competitive advantage.
References:
- “Artificial Intelligence in the Enterprise: AI Adoption and Trends by Industry” – Forbes
- “AI in the Workplace: Takeover or Collaboration?” – Deloitte
- “Ethics of Artificial Intelligence and Robotics” – Stanford Encyclopedia of Philosophy
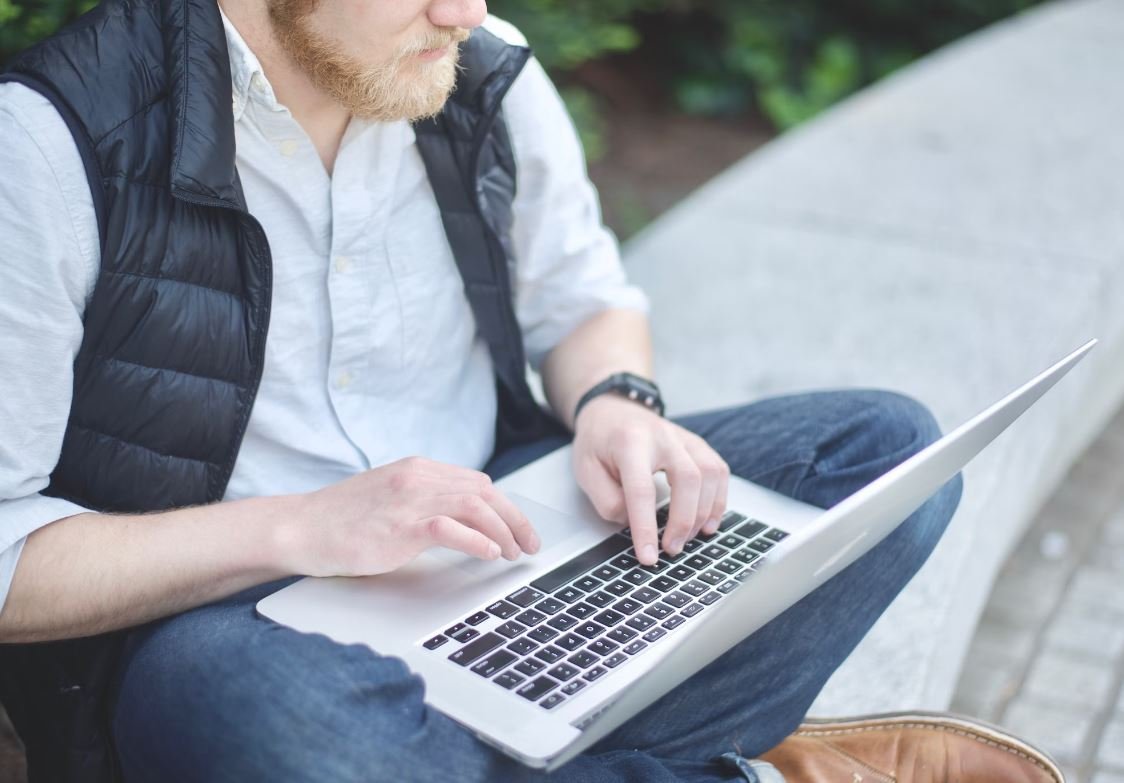
Common Misconceptions
Misconception 1: AI Enterprise Workflow is Completely Automated
One of the common misconceptions about AI Enterprise Workflow is that it is entirely automated, with minimal human intervention required. However, this is not entirely true. While AI technology plays a significant role in streamlining and optimizing workflows, human input is still crucial for decision-making, problem-solving, and monitoring the AI systems.
- AI workflows still require human expertise to set up and configure.
- Human intervention is needed in case of exception handling or unexpected situations.
- Ongoing human supervision is necessary to ensure the AI algorithms are achieving the desired results.
Misconception 2: AI Enterprise Workflow Replaces Humans
Another common misconception is that AI Enterprise Workflow aims to replace human workers entirely. While AI technology can automate repetitive and mundane tasks, its goal is to augment human capabilities rather than replacing them altogether. It allows employees to focus on more complex and value-added activities, thereby increasing overall productivity and efficiency.
- AI frees up human resources to engage in creative problem-solving and innovation.
- Human intuition and emotional intelligence are still vital for tasks such as customer interaction and decision-making.
- AI and humans can work collaboratively, with AI providing insights and recommendations, and humans making the final decisions.
Misconception 3: AI Enterprise Workflow is Expensive and Complex
Some people believe that implementing AI Enterprise Workflow is only viable for large organizations with abundant financial resources. However, this is not necessarily true. While implementing AI technology may require an initial investment, advancements in AI infrastructure and cloud computing have made it more accessible and scalable, even for smaller businesses.
- Cloud-based AI platforms offer affordable alternatives to on-premise solutions.
- AI application development is becoming easier with the availability of frameworks and tools.
- Consulting services and partnerships can help organizations navigate the complexity of AI implementation.
Misconception 4: AI Enterprise Workflow is a One-Size-Fits-All Solution
AI Enterprise Workflow is often mistakenly seen as a generic solution that can be applied universally across all industries and sectors. However, the reality is that each organization has unique challenges and requirements that may demand customizations and adaptations of AI technologies to suit their specific needs.
- AI systems need to be trained and fine-tuned for specific domains and data sets.
- Consideration of industry-specific regulations and ethical implications is crucial.
- Collaboration between data scientists and domain experts is necessary for effective customization.
Misconception 5: AI Enterprise Workflow is Infallible
Another misconception is that once an AI Enterprise Workflow is implemented, it will deliver flawless results and eliminate any possibility of errors or biases. However, like any other technology, AI systems are not perfect, and they are susceptible to biases, data limitations, and occasional errors.
- AI models can amplify existing biases present in the training data.
- Ongoing monitoring and auditing of AI systems are necessary to ensure fairness and accuracy.
- Regular updates and model retraining are required to adapt to changing data patterns and business needs.
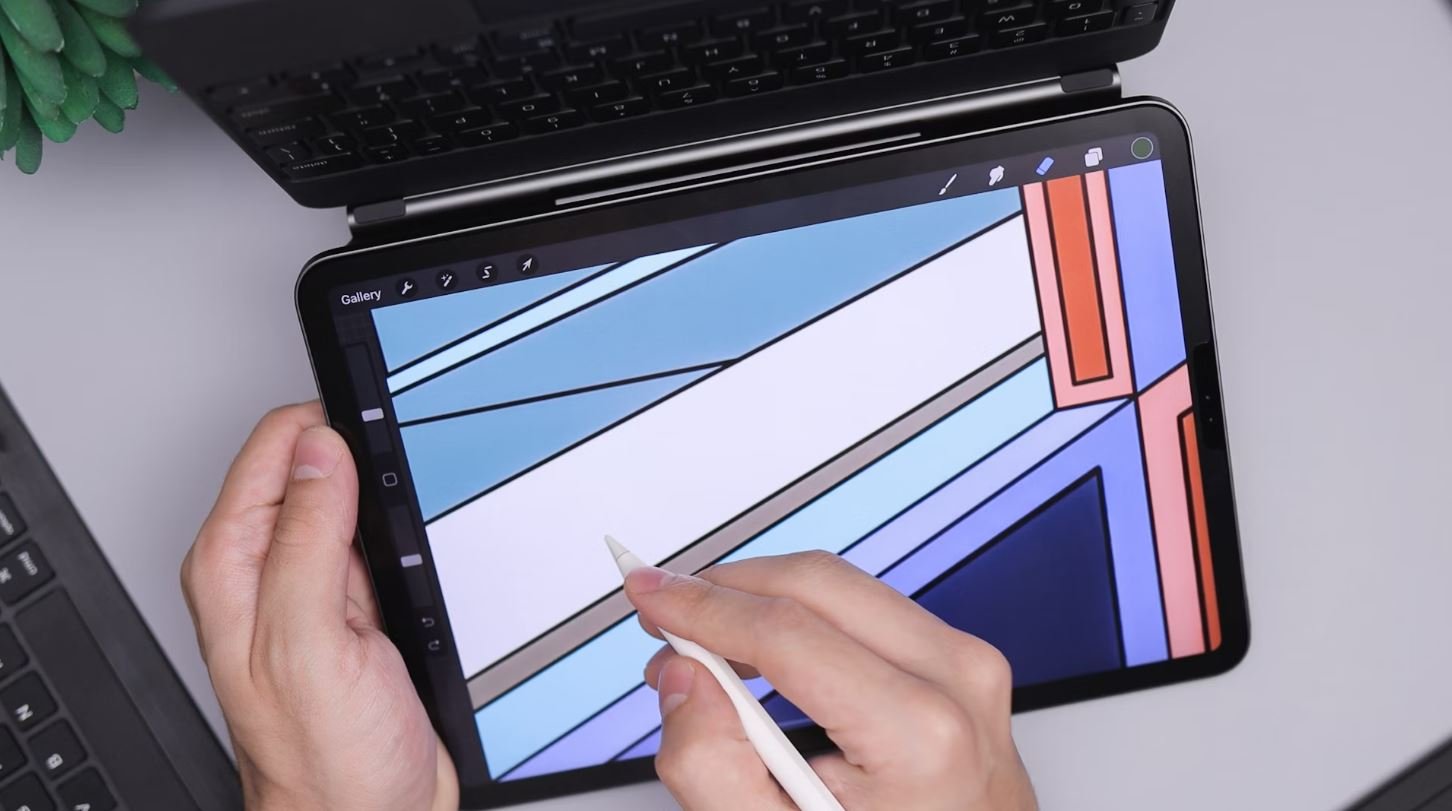
Introduction
Artificial intelligence (AI) has revolutionized the way enterprises operate by streamlining workflows and enhancing efficiency. This article explores various aspects of the AI enterprise workflow, including data collection, machine learning models, and decision-making processes. Through a series of compelling tables, you will gain insights into different stages of the workflow and witness the impact of AI on business operations.
1. Data Collection Methods
Table showcasing the different methods used for data collection in AI workflows, such as surveys, sensors, and web scraping.
Data Collection Method | Pros | Cons |
---|---|---|
Surveys | Quick and captures subjective data | Potential biases and response errors |
Sensors | Accurate and continuous data collection | High implementation costs |
Web Scraping | Large-scale data extraction | Legal and ethical considerations |
2. Popular AI Models
Dive into the world of AI models by examining popular ones broadly adopted across enterprises.
AI Model | Function | Benefits |
---|---|---|
Recurrent Neural Networks (RNN) | Sequences and time series analysis | Handles sequential data effectively |
Convolutional Neural Networks (CNN) | Image recognition and computer vision | Extracts features efficiently from visual data |
Generative Adversarial Networks (GAN) | Image and content generation | Produces realistic and innovative output |
3. AI in Decision-Making
Uncover how AI contributes to informed decision-making and its impact on business outcomes.
AI Decision-Making Process | Advantages | Limitations |
---|---|---|
Data Gathering and Analysis | Efficient processing of vast data | Lack of human intuition and context |
Predictive Modeling | Accurate forecasting and trend identification | Relies on historical data, susceptible to biases |
Scenario Simulation | Minimizes risk through comprehensive testing | May overlook unforeseen variables |
4. AI Deployment Strategies
Explore different strategies for deploying AI within an enterprise and their benefits.
Deployment Strategy | Advantages |
---|---|
Cloud-based AI | Scalability and cost-effectiveness |
On-Premises AI | Enhanced security and control |
Hybrid AI | Flexible deployment options |
5. AI Impact on Workforce
Analyze the effects of AI on the workforce, including job displacements and new roles.
Workforce Impact | Statistics |
---|---|
Job Displacements | 20% of jobs predicted to be automated by 2030 |
New Roles | 1.7 million new jobs expected to be created in AI field by 2030 |
6. Industries Embracing AI
Identify various industries that are wholeheartedly adopting AI technologies to improve their workflows.
Industry | AI Implementation |
---|---|
Healthcare | AI-assisted diagnostics, personalized medicine |
Retail | Inventory management, personalized recommendations |
Manufacturing | Quality control, predictive maintenance |
7. AI Ethics and Regulations
Explore the ethical considerations and regulatory frameworks surrounding AI adoption.
Ethical Considerations | Regulatory Frameworks |
---|---|
Fairness and bias | General Data Protection Regulation (GDPR) |
Transparency and explainability | Ethical Guidelines for Trustworthy AI by European Commission |
Accountability and responsibility | AI Principles by the Organisation for Economic Co-operation and Development (OECD) |
8. AI ROI Statistics
Present the return on investment (ROI) statistics associated with AI implementation.
Industry | Median ROI |
---|---|
Finance | 58% |
Transportation | 71% |
Telecommunications | 63% |
9. AI and Customer Satisfaction
Examine the impact of AI on customer satisfaction and loyalty in various industries.
Industry | Customer Satisfaction Improvement |
---|---|
Hospitality | 15% increase in personalized service |
E-commerce | 12% reduction in customer complaints |
Banking | 10% higher customer retention |
Conclusion
In this AI enterprise workflow exploration, we delved into the various components of AI adoption, including data collection methods, popular AI models, decision-making processes, deployment strategies, workforce impact, industries embracing AI, ethical considerations, ROI statistics, and customer satisfaction enhancements. The tables presented captivating insights that demonstrate the transformative power of AI across sectors. As enterprises continue to integrate AI technologies, it is imperative to address ethical concerns while leveraging the enormous potential for improved efficiency, accuracy, and customer satisfaction. Embracing AI in the workflow will undoubtedly pave the way for a brighter future in enterprise operations.
Frequently Asked Questions
AI Enterprise Workflow
What is an AI enterprise workflow?
Why is an AI enterprise workflow important?
What are the key components of an AI enterprise workflow?
How does an AI enterprise workflow impact business operations?
What challenges can arise in implementing an AI enterprise workflow?
How can organizations ensure data privacy and security in their AI enterprise workflow?
What role does collaboration play in an AI enterprise workflow?
How can AI enterprise workflows be continuously improved?
Are there any industry standards or frameworks for AI enterprise workflows?
Is an AI enterprise workflow applicable to all types of businesses?