Introduction
Artificial Intelligence (AI) technology has made significant advancements in recent years, revolutionizing various industries and sectors, including education. AI tools and learning models are transforming the way we teach and learn, providing personalized experiences, improving outcomes, and fostering a deeper understanding of complex concepts. This article explores how AI tools and learning models are revolutionizing education, the benefits they offer, and the future implications of these advancements.
Key Takeaways:
– AI tools and learning models are revolutionizing education.
– They offer personalized learning experiences.
– AI improves educational outcomes across different fields.
– Predictive analytics and data insights help educators make informed decisions.
– AI technology enables remote learning and accessibility.
Personalized Learning Experiences
One of the most significant advantages of using AI tools and learning models in education is the ability to personalize learning experiences. AI algorithms analyze vast amounts of data to understand individual learning patterns, preferences, and strengths, enabling the development of customized learning paths. **With the help of AI, educators can tailor instruction to address specific needs, ensuring students receive the most appropriate support and guidance.** This personalized approach enhances engagement, motivation, and overall learning outcomes.
Improving Educational Outcomes
AI tools and learning models have the potential to significantly improve educational outcomes across various fields. By adapting to individual student needs, AI-driven systems can identify knowledge gaps and provide targeted interventions to bridge them. **This individualized attention helps students grasp difficult concepts more effectively** and promotes a deeper and more meaningful understanding of the subject matter. Moreover, AI-powered systems can provide immediate feedback on assignments and assessments, enabling students to make necessary revisions and improvements in real-time, fostering continuous learning and growth.
Predictive Analytics and Data Insights
AI technology also enables educators to make more informed decisions through predictive analytics and data insights. By analyzing student data, AI algorithms can identify patterns and trends, helping educators understand the effectiveness of teaching methods, curriculum design, and assessment strategies. **These data insights enable educators to make data-driven decisions** regarding curriculum modifications, support interventions, and resource allocation, resulting in more targeted and impactful instruction.
Table 1: Potential Impact of AI in Education
| Impact | Description |
|——–|————-|
| Personalized | AI offers personalized learning experiences tailored to individual student needs. |
| Learning | This approach improves engagement, motivation, and overall learning outcomes. |
| Outcomes | |
| Improved | AI tools adapt to individual student needs, identifying knowledge gaps and providing targeted interventions. |
| Education | This personalized attention promotes a deeper understanding of subjects. |
| Outcomes | |
| Enhanced | AI provides immediate feedback on assignments and assessments, fostering continuous learning and growth. |
| Feedback | |
Remote Learning and Accessibility
Another remarkable benefit of AI tools and learning models is the enhancement of remote learning and accessibility. AI technology enables the creation of virtual learning environments, simulations, and interactive modules that simulate real-world scenarios. **This immersive learning experience transcends physical limitations,** allowing students to explore and understand complex concepts in a safe and engaging manner. Additionally, AI-powered chatbots and tutoring systems provide instant support and guidance to students, even in the absence of face-to-face interaction, fostering inclusivity and equal access to education.
Table 2: The Evolution of AI in Education
| Era | Technology | Description |
|————–|—————————|——————————–|
| Foundational | Expert Systems | Rule-based systems providing expert knowledge. |
| Stage | | |
| Enhancement | Intelligent Tutoring Systems | Early AI systems delivering personalized instruction. |
| Stage | | |
| Transformation | Adaptive Learning Systems | AI-driven systems adapting to individual student needs. |
| Stage | | |
| Expansion | Virtual Reality (VR) | Immersive environments enhancing learning experiences. |
| Stage | | |
| Future | Natural Language | AI systems understanding and responding to human language. |
| | Processing (NLP) | |
Future Implications
The future implications of AI tools and learning models in education are vast. As AI technology continues to evolve, we can expect further advancements in pedagogy, curriculum design, and student support systems. *AI may enable hyper-personalized learning experiences where learning materials are dynamically created as per student’s real-time needs.* Additionally, AI can provide valuable career guidance by analyzing individual strengths, aspirations, and labor market demands, helping students make informed choices about their educational and professional paths.
Table 3: AI Adoption in Education Institutions
| Adoption | Description |
|———-|—————————————————————————–|
| Early | AI adoption is in its early stages across educational institutions worldwide. |
| Stages | Educators are exploring and implementing AI tools for specific applications. |
| | |
| Partial | Some institutions have partially integrated AI to enhance teaching and learning. |
| Adoption | These initiatives focus on specific subjects, grade levels, or support services. |
| | |
| Widespread | As AI technology matures, we can expect its widespread integration across educational institutions. |
| Adoption | This integration will be integral to meet the evolving demands of students and educators. |
In conclusion, AI tools and learning models are revolutionizing education by providing personalized learning experiences, improving educational outcomes, and enabling data-driven decision-making in teaching. Additionally, the adoption of AI technology facilitates remote learning and ensures better accessibility to education. With future advancements on the horizon, AI has the potential to significantly transform the landscape of education, empowering both students and educators on their continuous learning journey.

Common Misconceptions
Misconception: AI tools can replace humans
One common misconception people have about AI tools is that they can fully replace human involvement. While AI tools are capable of automating certain tasks and making processes more efficient, they still require human guidance and oversight.
- AI tools are designed to assist humans, not replace them.
- Human judgment and decision-making are crucial in complex situations that AI may struggle to navigate.
- AI tools can enhance human productivity and performance, but they cannot completely substitute for human capabilities.
Misconception: All AI learning models are unbiased
Another misconception is that AI learning models are inherently unbiased. However, AI models are only as good as the data they are trained on, and if the data itself is biased, it can result in biased outcomes.
- Data used to train AI models can be influenced by human biases and prejudices.
- AI models can unintentionally perpetuate or amplify existing societal biases.
- Continual monitoring and evaluation of AI models are necessary to identify and address biases.
Misconception: AI tools and learning models are infallible
There is often a belief that AI tools and learning models are infallible and always provide correct results. However, AI systems can still make mistakes and errors, just like any technology.
- AI models can produce inaccurate results when faced with novel or unfamiliar situations.
- Incorrect or biased inputs can lead to flawed outcomes.
- Regular testing and validation are necessary to ensure the reliability and accuracy of AI tools.
Misconception: AI tools can think and learn like humans
Many people assume that AI tools can think and learn just like humans do. However, AI models operate based on algorithms and patterns, rather than true understanding and consciousness.
- AI tools operate based on pre-defined rules and algorithms.
- AI models lack subjective experiences and emotions that are intrinsic to human thinking.
- AI tools require significant amounts of data to “learn,” unlike humans who can learn from a few examples.
Misconception: AI tools eliminate the need for specialized human skills
Some individuals wrongly believe that AI tools eliminate the need for specialized human skills, rendering certain professions and expertise obsolete. However, AI tools are designed to complement and augment human abilities, rather than replace them entirely.
- Specialized human skills are still crucial for interpreting, validating, and utilizing AI-generated insights.
- AI tools require trained human operators to set objectives, interpret results, and make informed decisions.
- Collaboration between AI tools and human professionals leads to improved outcomes in various fields.
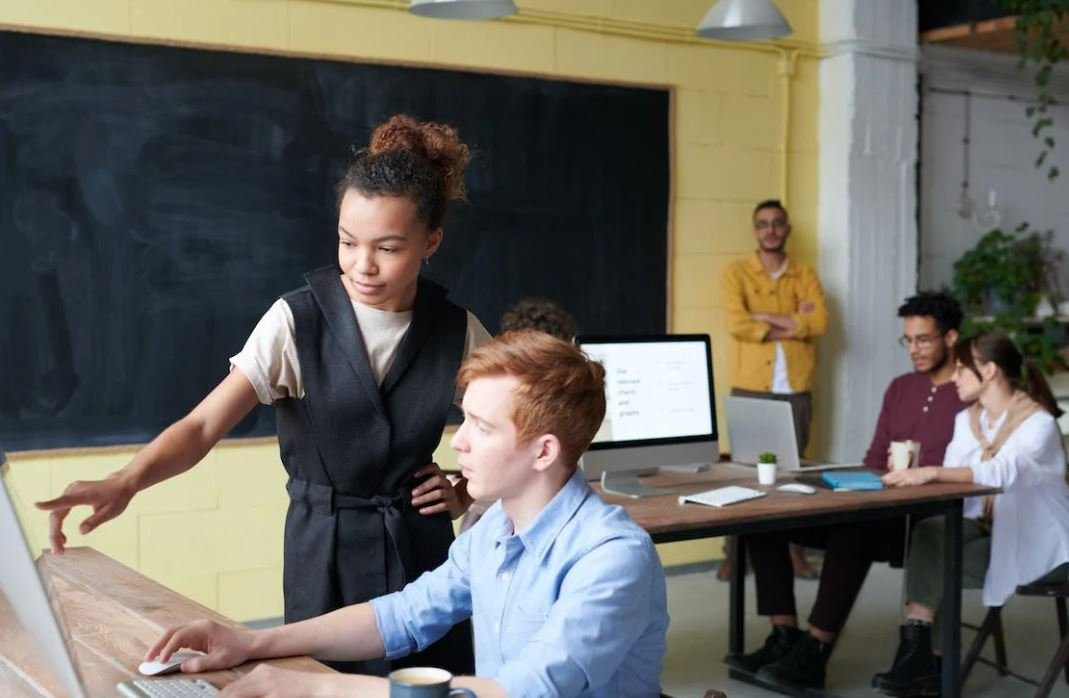
Artificial Intelligence in Healthcare
Artificial intelligence (AI) has made remarkable advancements in various industries, including healthcare. AI tools and learning models are revolutionizing the way healthcare professionals diagnose, treat, and manage medical conditions. The following tables provide an intriguing glimpse into the impact and potential of AI in the healthcare field.
Table: AI Applications in Medical Imaging
AI has significantly improved medical imaging techniques, aiding healthcare providers in accurate diagnoses. The table below highlights some AI applications in medical imaging.
AI Application | Description |
---|---|
CAD Systems | Computer-aided detection and diagnosis systems that help identify abnormalities in medical images. |
Radiology Automation | AI algorithms that automate radiology processes, reducing human error and improving efficiency. |
Automated Tumor Segmentation | AI models that accurately segment tumors in medical images, aiding in treatment planning. |
Table: AI Chatbots in Patient Care
AI chatbots provide virtual assistance to patients, enhancing healthcare accessibility and reducing workload on medical staff. The table below presents various applications of AI chatbots in patient care.
AI Chatbot | Function |
---|---|
Virtual Receptionist | Assists patients with appointment scheduling, prescription refills, and general inquiries. |
Symptom Checker | Helps users determine potential illnesses based on symptoms and provides appropriate recommendations. |
Mental Health Support | Offers accessible mental health support and resources, promoting well-being. |
Table: AI in Drug Discovery
AI’s ability to analyze vast amounts of data and predict outcomes efficiently makes it valuable in drug discovery research. The table below showcases some AI applications in this field.
AI Application | Purpose |
---|---|
Target Identification | Identifies potential targets for new therapies based on molecular data analysis. |
Molecule Generation | Generates novel molecules with desirable properties, aiding in drug development. |
Compound Screening | Analyzes large compound libraries to identify molecules with high medicinal potential. |
Table: AI-Enhanced Robotic Surgery
AI technology has revolutionized the field of robotic surgery, improving precision and reducing complications. The table below highlights some AI applications in this area.
AI Application | Description |
---|---|
Image-Guided Surgery | Provides real-time guidance to surgeons during procedures, enhancing accuracy. |
Surgeon Augmentation | Assists surgeons by analyzing preoperative data, suggesting optimal surgical approaches. |
Smart Instrumentation | Enables instruments to adapt to the surgical environment, reducing tissue damage. |
Table: AI-Enabled Personalized Medicine
AI allows healthcare providers to tailor treatment plans based on an individual’s unique characteristics and medical history. The table below illustrates different AI-enabled applications for personalized medicine.
AI Application | Function |
---|---|
Genetic Profiling | Analyzes genetic information to identify personalized treatment options. |
Medication Optimization | Creates personalized drug regimens based on patient-specific factors. |
Disease Risk Assessment | Uses algorithms to predict an individual’s likelihood of developing certain conditions. |
Table: AI-Assisted Patient Monitoring
AI enables continuous patient monitoring, improving early detection of complications and enhancing patient outcomes. The table below presents various AI-assisted patient monitoring solutions.
AI Solution | Function |
---|---|
Wearable Devices | Collects real-time health data, such as heart rate and sleep patterns, for remote monitoring. |
Anomaly Detection | Utilizes machine learning algorithms to identify abnormal patterns in physiological data. |
Early Warning Systems | Alerts healthcare providers of potential deterioration in a patient’s condition. |
Table: AI in Precision Oncology
AI offers promising solutions to improve cancer treatment and outcomes through precision oncology approaches. The table below showcases various AI applications in precision oncology.
AI Application | Purpose |
---|---|
Tumor Classification | Classifies tumors into subtypes for tailored treatment decisions. |
Drug Response Prediction | Predicts a patient’s response to specific cancer treatments, optimizing therapy choices. |
Prognostic Tools | Assesses patient outcomes based on various clinical and genetic factors. |
Table: AI-Based Patient Risk Prediction
AI algorithms analyze patient data to predict the risk of developing certain diseases or complications, empowering healthcare professionals to take preventive measures. The table below presents some AI-based patient risk prediction applications.
AI Application | Description |
---|---|
Cardiovascular Risk Prediction | Utilizes machine learning to estimate a patient’s risk of cardiovascular events. |
Diabetes Risk Assessment | Predicts the likelihood of an individual developing diabetes based on various factors. |
Patient Deterioration Prediction | Analyzes patient data in real-time to forecast deterioration and enable proactive interventions. |
Table: AI-Driven Electronic Health Records (EHR)
AI assists in streamlining electronic health records (EHR) management, providing quick access to relevant patient information and reducing administrative burdens. The table below illustrates AI-driven EHR capabilities.
AI Capability | Function |
---|---|
Natural Language Processing | Extracts relevant information from unstructured EHR data, improving data analysis. |
Clinical Decision Support | Suggests treatment options and alerts healthcare professionals to potential errors or drug interactions. |
Patient Profile Dashboard | Provides a comprehensive view of patient data, enabling efficient care coordination. |
Conclusion
The integration of AI tools and learning models into healthcare has opened up a world of possibilities. From enhancing medical imaging diagnosis to personalizing treatment plans, AI is transforming patient care. By leveraging the power of AI, healthcare professionals have the opportunity to improve outcomes, reduce costs, and save lives. As technology continues to advance, the ongoing collaboration between AI and healthcare holds great promise for the future of medicine.
Frequently Asked Questions
AI Tools and Learning Models
Q: What are AI tools?
A: AI tools are software applications or programs that utilize artificial intelligence techniques to perform specific tasks.
Q: What is a learning model in AI?
A: A learning model in AI refers to an algorithm or mathematical function that is designed to learn from data.
Q: How do AI tools improve efficiency?
A: AI tools can improve efficiency by automating tasks, reducing human error, and analyzing large amounts of data.
Q: What are the benefits of using AI tools in business?
A: Some benefits of using AI tools in business include improved customer service, increased operational efficiency, better decision-making, and enhanced personalization.
Q: What are the limitations of AI tools?
A: Some limitations of AI tools include data dependency, potential bias, the need for continuous updating, and ethical considerations.
Q: How can AI tools be integrated into existing systems?
A: AI tools can be integrated into existing systems through APIs or AI-powered software development frameworks.
Q: What is the difference between supervised and unsupervised learning?
A: Supervised learning involves labeled training data, while unsupervised learning works with unlabeled data.
Q: What is reinforcement learning and how does it work?
A: Reinforcement learning is a type of machine learning where an agent learns through trial and error by interacting with an environment.
Q: Are AI tools and learning models capable of self-improvement?
A: Some AI tools and learning models are designed to be self-improving and can adapt based on new data or user interactions.
Q: How can I ensure ethical use of AI tools and learning models?
A: To ensure ethical use, organizations should consider factors such as data privacy, transparency, fairness, and accountability.