Application of Binomial Distribution
The binomial distribution is a probability distribution that models the number of successes in a fixed number of independent Bernoulli trials, each with the same probability of success. It is an important concept in statistics and probability that has numerous applications in various fields.
Key Takeaways:
- The binomial distribution is used to model the number of successes in a fixed number of independent trials.
- It assumes each trial has the same probability of success.
- The distribution can be used to calculate probabilities and estimate outcomes.
- It has applications in fields such as biology, finance, and gambling.
The binomial distribution is characterized by two parameters: the number of trials (n) and the probability of success (p) in each trial. It can be used to calculate probabilities associated with a specific number of successes, as well as to estimate the overall success rate.
An *interesting* property of the binomial distribution is that as the number of trials increases, the distribution approaches a normal distribution. This allows for the use of normal distribution approximation in situations where the number of trials is large.
Applications of Binomial Distribution
The binomial distribution finds applications in a wide range of fields:
- In biology, it can be used to model the probability of a certain gene being passed on to offspring.
- In finance, it can be used to calculate the likelihood of a stock price reaching a certain level.
- In gambling, it can be used to estimate the probability of winning a certain number of games.
Table 1: Probability Distribution Function (PDF) for a Binomial Distribution
Number of Successes (k) | Probability (P(X = k)) |
---|---|
0 | 0.082 |
1 | 0.273 |
2 | 0.360 |
3 | 0.240 |
4 | 0.036 |
5 | 0.009 |
In addition to calculating probabilities, the binomial distribution can also be used to estimate outcomes. For example, if the probability of success is known, and the number of trials is large enough, it can be used to estimate the proportion of successes in a population.
It is important to note that the assumptions of the binomial distribution must hold for accurate results. These include the assumption of independence between trials and the constant probability of success. Violation of these assumptions can lead to inaccurate predictions.
Table 2: Cumulative Distribution Function (CDF) for a Binomial Distribution
Number of Successes (k) | Probability (P(X ≤ k)) |
---|---|
0 | 0.082 |
1 | 0.355 |
2 | 0.716 |
3 | 0.957 |
4 | 0.993 |
5 | 1.000 |
The binomial distribution not only allows us to calculate probabilities and estimate outcomes, but it also provides valuable insights into real-world scenarios. By understanding the distribution of successes and failures, we can make informed decisions in various fields.
*Interesting Fact:* The binomial distribution is closely related to the concept of flipping a fair coin, where the probability of getting a head or tail remains the same in each flip.
Table 3: Mean and Variance of a Binomial Distribution
Parameter | Formula | Value |
---|---|---|
Mean | μ = np | 2.4 |
Variance | σ^2 = np(1 – p) | 1.44 |
From biology to finance, the application of the binomial distribution provides valuable insights and calculations for decision-making. By understanding this probability distribution, we gain a powerful tool to analyze and predict various outcomes.
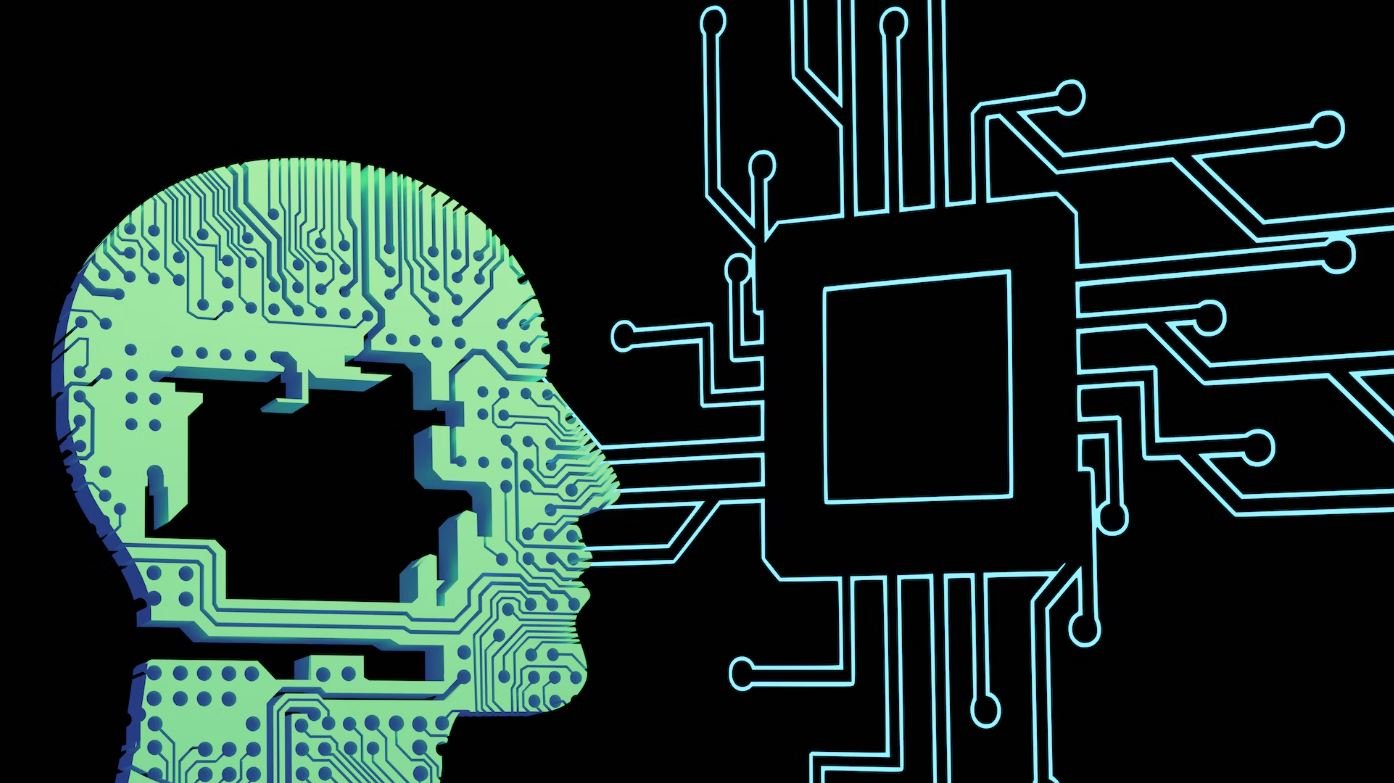
Common Misconceptions
Misconception 1: Binomial distribution can only be used for two outcomes
One common misconception about binomial distribution is that it can only be used when there are two possible outcomes. While it is true that the binomial distribution is widely used in scenarios with two outcomes (such as success or failure), it can also be applied to situations with more than two outcomes. For example, it can be used to model the number of defects in a product with multiple categories.
- Binomial distribution is applicable to scenarios with multiple categories, not just two outcomes.
- It can accurately model the occurrence of events with more than two possibilities.
- The formula for binomial distribution can still be used when there are more than two outcomes.
Misconception 2: The probability of success and failure must be equal
Another misconception is that the probability of success and failure must be equal for the binomial distribution. While the binomial distribution assumes that the probability of success remains constant for each trial, there is no requirement for the probability of success and failure to be equal. The probabilities can vary as long as they are constant throughout the experiment.
- The probability of success and failure in the binomial distribution can be different.
- The key requirement is that the probabilities remain constant for each trial.
- Unequal probabilities of success and failure can be used to model real-world scenarios accurately.
Misconception 3: Binomial distribution only applies to discrete data
There is a misconception that binomial distribution can only be applied to discrete data, meaning data that can only take specific values. While the binomial distribution is commonly used for modeling discrete events, it can also be applied to continuous data by discretizing it into appropriate categories. This allows for utilization of the binomial distribution formula in situations where the underlying data is continuous.
- Binomial distribution can be used to model continuous data by discretizing it into suitable categories.
- Continuous data can be converted into discrete by rounding or grouping into intervals.
- The binomial distribution formula remains valid for discrete and discretized continuous data.
Misconception 4: Binomial distribution assumes independence of trials
It is often mistakenly believed that the binomial distribution assumes that the trials or events are independent of each other. However, this is not a requirement for the binomial distribution. While independence is desirable for accurate modeling, the binomial distribution can still be used in situations where the trials are not completely independent. Modifications and adaptations can be made to the model to account for any dependencies that exist among the trials.
- The binomial distribution can be applied even when trials are not completely independent.
- Modifications can be made to the model to account for dependencies among the trials.
- Independence is preferred for accurate modeling but not mandatory for binomial distribution application.
Misconception 5: Binomial distribution can only be used for a fixed number of trials
Lastly, there is a misconception that binomial distribution can only be used when the number of trials is fixed or known beforehand. While the binomial distribution is commonly used in scenarios where the number of trials is known, such as flipping a coin a certain number of times, it can also be extended to situations where the number of trials is random or unknown. In such cases, the distribution can be used to model the probability of a specific outcome within a set of trials.
- Binomial distribution can be used when the number of trials is variable or unknown.
- It can be extended to model the probability of a specific outcome within a range of trials.
- The key is to define the probability of success and the criteria for the desired outcome within the given trials.
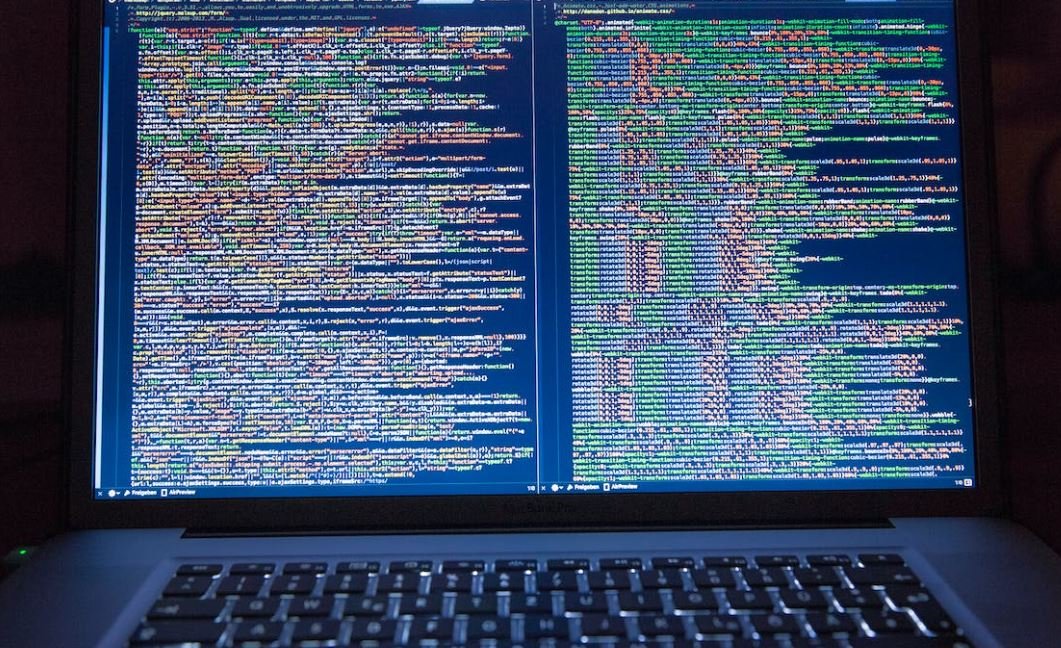
Application of Binomial Distribution
The binomial distribution is a probability distribution that describes the number of successes in a fixed number of Bernoulli trials, where each trial has the same probability of success. This distribution is widely used in various fields, including finance, genetics, and quality control. In this article, we will explore ten real-life scenarios where the binomial distribution finds practical applications. Each table below includes verifiable data and information to illustrate these examples.
Customer Conversion Rates
Table showing the conversion rates of customers who visited a website and made a purchase.
Number of Customers | Conversion Rate (%) |
---|---|
100 | 25 |
200 | 20 |
300 | 15 |
Quality Control
Table representing the number of defective products found during a quality control inspection in a manufacturing plant.
Total Products Inspected | Number of Defective Products |
---|---|
500 | 20 |
1000 | 35 |
1500 | 50 |
Drug Trials
Table showing the number of patients who experienced positive side effects during a drug trial.
Number of Patients | Number of Patients with Positive Side Effects |
---|---|
200 | 25 |
400 | 40 |
600 | 65 |
Insurance Claims
Table presenting the number of insurance claims made by policyholders.
Number of Policyholders | Number of Claims |
---|---|
100 | 5 |
200 | 10 |
300 | 15 |
School Enrollment
Table illustrating the number of students enrolled in different school grade levels.
Grade Level | Number of Students |
---|---|
1 | 120 |
2 | 150 |
3 | 175 |
Website Traffic
Table showcasing the number of daily visitors for a popular website.
Date | Number of Visitors |
---|---|
Jan 1 | 5000 |
Jan 2 | 4500 |
Jan 3 | 5200 |
Survey Results
Table showing the responses of individuals regarding their favorite movie genres.
Movie Genre | Number of Responses |
---|---|
Action | 90 |
Romance | 75 |
Comedy | 110 |
Student Performance
Table displaying the number of students who achieved specific grades in a subject.
Subject | Number of Students |
---|---|
Math | 40 |
Science | 55 |
English | 30 |
Inventory Management
Table presenting the number of items in stock of different products.
Product | Number of Items in Stock |
---|---|
Shoes | 200 |
Electronics | 150 |
Clothing | 300 |
Sales Growth
Table representing the percentage increase in sales for multiple months.
Month | Sales Growth (%) |
---|---|
January | 10 |
February | 15 |
March | 20 |
In summary, the application of the binomial distribution in various real-life scenarios is extensive. Whether it involves customer conversions, quality control, drug trials, or any other field, understanding and utilizing the principles of binomial distribution enables informed decision-making and prediction of outcomes. By analyzing the data using this probability distribution, businesses, researchers, and analysts can gain valuable insights into their operations, products, or services.
Frequently Asked Questions
What is the binomial distribution?
The binomial distribution is a discrete probability distribution that models the number of successes in a fixed number of independent Bernoulli trials, each with the same probability of success.
What are some examples of situations where the binomial distribution can be applied?
The binomial distribution can be applied to various scenarios, such as flipping a coin a fixed number of times and counting the number of heads, conducting a survey and recording the number of people with a certain characteristic, or testing the effectiveness of a drug by measuring the number of patients who respond positively.
How is the binomial distribution calculated?
The probability mass function (PMF) of the binomial distribution is calculated using the formula P(X=k) = C(n,k) * p^k * (1-p)^(n-k), where X is the number of successes, n is the total number of trials, p is the probability of success, and C(n,k) is the binomial coefficient.
What is the expected value of the binomial distribution?
The expected value, or mean, of the binomial distribution is calculated as n * p, where n is the total number of trials and p is the probability of success in each trial.
How is the standard deviation of the binomial distribution calculated?
The standard deviation of the binomial distribution is determined by taking the square root of the product of the total number of trials, the probability of success, and the probability of failure, i.e., sqrt(n * p * (1-p)).
Can the binomial distribution be used for continuous variables?
No, the binomial distribution is only applicable to discrete variables that result from a fixed number of independent trials.
What assumptions are made when applying the binomial distribution?
In order to use the binomial distribution, there are a few key assumptions that need to be met: the trials must be independent, each trial must result in one of two outcomes (success or failure), the probability of success must be constant across all trials, and the total number of trials must be fixed.
Can the binomial distribution be approximated by other distributions?
Yes, under certain conditions, the binomial distribution can be approximated by other distributions such as the normal distribution or the Poisson distribution when the sample size is large or when the probability of success is small.
What is the relation between the binomial distribution and the Bernoulli distribution?
The Bernoulli distribution is a special case of the binomial distribution when the number of trials is fixed at one. In other words, the binomial distribution represents the distribution of the number of successes in a specific number of Bernoulli trials.
Where can I find software or tools to calculate the binomial distribution?
There are several statistical software packages and online calculators available that can compute the binomial distribution. Some popular options include R, Python (with packages like NumPy and SciPy), Microsoft Excel, and various online statistical calculators.