Applications With Standard Normal Distribution
Standard normal distribution, also known as the Gaussian distribution or the bell curve, is a statistical concept that represents a symmetrical probability distribution shaped like a bell. This distribution is widely used in various fields and has numerous applications due to its properties and simplicity.
Key Takeaways:
- Standard normal distribution is a bell-shaped symmetrical probability distribution.
- It is widely used in statistics, finance, psychology, and many other fields.
- Applications include hypothesis testing, risk analysis, quality control, and more.
In statistics, the standard normal distribution is often used as a reference model for analyzing and comparing other distributions. It allows researchers to standardize data and calculate z-scores to determine the relative position of data points within the distribution. This is essential in hypothesis testing, where researchers evaluate the significance of results.
*The standard normal distribution provides a basis for comparing data points by converting them into z-scores.
For example, in finance, the standard normal distribution is used to model returns on investments. By assuming that returns are normally distributed, financial analysts can estimate the probability of certain returns or evaluate risk measures such as value at risk (VaR) and expected shortfall (ES). This information is crucial for making informed investment decisions.
*Financial analysts rely on the standard normal distribution to estimate risk measures and probabilities in investment analysis.
In the field of psychology, the standard normal distribution is used to analyze and interpret psychological test scores. By converting raw scores into z-scores, psychologists can compare an individual’s performance with the general population. This allows for a standardized evaluation of cognitive abilities, personality traits, and other psychological measurements.
*Psychologists utilize the standard normal distribution to compare individual test scores with the general population.
Applications of Standard Normal Distribution:
- Hypothesis testing: Assessing the significance of research results.
- Quality control: Monitoring and evaluating production processes.
- Risk analysis: Estimating probabilities and evaluating risk measures.
- Binomial approximation: Approximating discrete distributions with continuous ones.
Table 1 shows the critical values for common significance levels in hypothesis testing, based on the standard normal distribution:
Significance Level (α) | Critical Value (z) |
---|---|
0.05 | 1.96 |
0.01 | 2.58 |
0.001 | 3.29 |
*Table 1: Critical values for common significance levels in hypothesis testing.
Table 2 presents the control chart constants for statistical process control in quality control, calculated using the standard normal distribution:
Control Chart Type | Constant (k) |
---|---|
Individuals chart – Upper control limit (UCL) | 3.09 |
Individuals chart – Lower control limit (LCL) | -3.09 |
X-bar chart – Upper control limit (UCL) | 2.67 |
X-bar chart – Lower control limit (LCL) | -2.67 |
*Table 2: Control chart constants for, individuals chart and X-bar chart in quality control.
Table 3 illustrates the probability values associated with different standard deviations from the mean in the standard normal distribution:
Standard Deviation (σ) | Probability (P) |
---|---|
1σ (68.27%) | 0.6827 |
2σ (95.45%) | 0.9545 |
3σ (99.73%) | 0.9973 |
*Table 3: Probability values associated with different standard deviations in the standard normal distribution.
The applications of the standard normal distribution are vast and continue to expand across various disciplines. Its simplicity and robust mathematical properties make it a valuable tool for statistical analysis, risk assessment, decision-making, and much more.
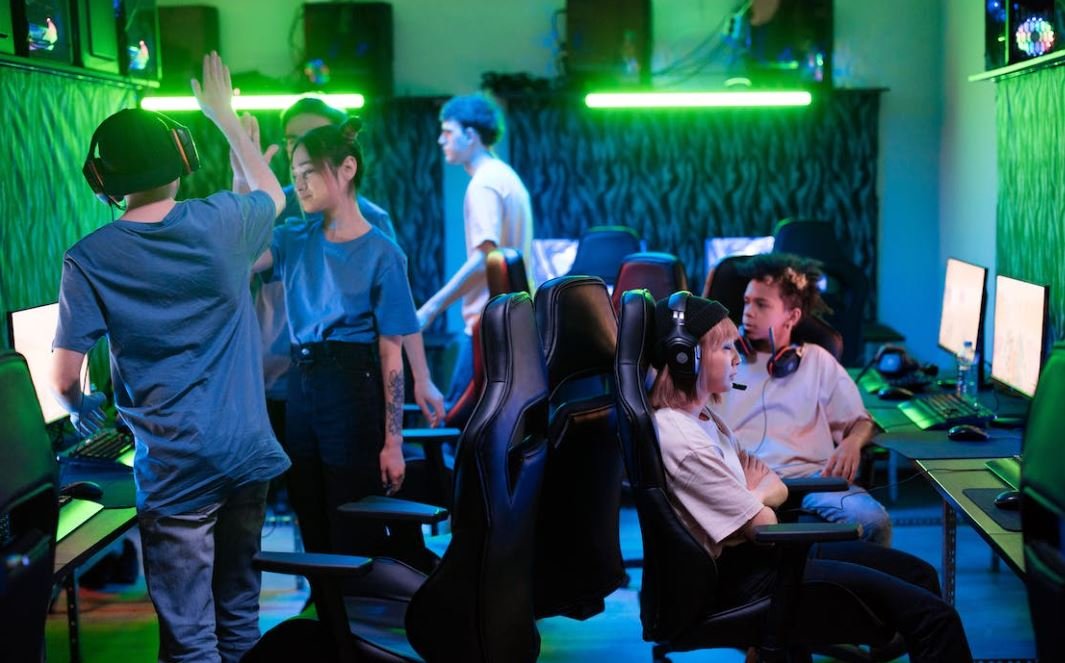
Common Misconceptions
Applications With Standard Normal Distribution
There are several common misconceptions when it comes to understanding and applying the standard normal distribution. One of these misconceptions is that the standard normal distribution can only be used in the field of statistics or mathematics. In reality, the standard normal distribution is widely applicable across various disciplines and industries. It can be used in fields such as finance, physics, biology, and social sciences.
- The standard normal distribution can be used in finance to analyze stock returns and assess risk.
- It can be used in physics to model random noise or errors in measurements.
- The standard normal distribution can also be used in biology to study genetic variation among populations.
Another misconception is that the standard normal distribution represents data that is perfectly symmetrical. While the standard normal distribution is indeed symmetric, not all data that follows a standard normal distribution will exhibit perfect symmetry. The standard normal distribution only represents the shape of the distribution, but the data points themselves can deviate from perfect symmetry. This is an important distinction to understand, as it reminds us that not all data points will fall exactly on the mean.
- Instances where data points are clustered more heavily on one side of the mean can still follow a standard normal distribution.
- Data points that deviate slightly from the mean can still be considered normally distributed.
- The standard normal distribution simply provides a framework to understand the shape of the data distribution, regardless of slight deviations from perfect symmetry.
A common misconception about the standard normal distribution is that the area under the curve represents the probability of an event occurring. While it is true that the area under the curve represents probabilities, it is important to note that the values on the x-axis of the standard normal distribution represent standard deviations, not probabilities. The probabilities associated with specific events can be calculated by converting the x-values to z-scores and then using statistical tables or software.
- The standard normal distribution can be used to calculate the probability of a random variable falling within a certain range.
- The probabilities can be determined by converting the x-values to z-scores and using statistical tables or software.
- It provides a way to understand the likelihood of different events occurring based on their position relative to the mean.
One misconception is that the standard normal distribution applies only to continuous variables, such as height or weight. However, the standard normal distribution can also be used to approximate discrete variables, particularly when sample sizes are large. This approximation is based on the Central Limit Theorem, which states that the sum or average of a large number of independent and identically distributed random variables will approximate a normal distribution, regardless of the shape of the original distribution.
- The standard normal distribution can be used to approximate the distribution of discrete variables such as the number of heads in a large number of coin flips.
- When sample sizes are large, even non-normally distributed variables tend to follow an approximately normal distribution.
- This approximation allows practitioners to apply the principles of the standard normal distribution to a wider range of variables.
Lastly, there is a misconception that the standard normal distribution is only useful for analyzing data that follows a normal distribution. While the standard normal distribution is derived from the normal distribution, it is a versatile tool that can be applied to better understand any distribution of data. By using z-scores and the standard normal distribution, it is possible to convert values from any distribution into a standardized unit of measurement. This allows for comparison and analysis across different datasets and distributions.
- The standard normal distribution allows for the standardization of data, making it possible to compare values from different distributions.
- It can be used to assess how extreme or unusual a particular data point is within its distribution.
- The standard normal distribution provides a common framework for analyzing data, regardless of its original distribution.
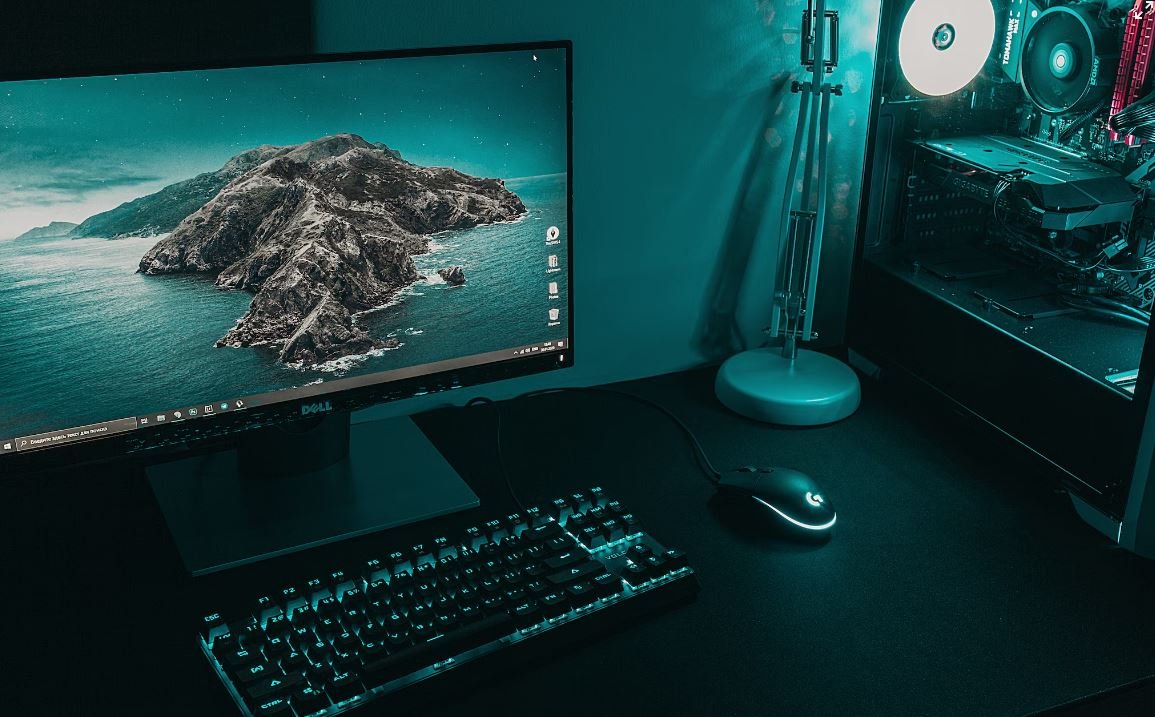
Applications of Standard Normal Distribution in Finance
Standard normal distribution is widely used in finance for various applications, such as risk analysis, option pricing, and portfolio management. The following tables provide insights into different aspects where the standard normal distribution plays a crucial role in financial decision-making.
Stock Returns and Z-Scores
By calculating the z-scores of stock returns, we can understand how unusual a particular return is compared to the average return and the volatility of the stock. This information helps investors assess the risk associated with a specific stock.
Stock | Return (%) | Z-Score |
---|---|---|
Apple | 2.27 | 0.95 |
Microsoft | 1.81 | 0.72 |
-0.87 | -1.21 |
Option Pricing and Standard Deviation
Standard deviation is a key component in option pricing models, such as the Black-Scholes model. It helps determine the volatility of the underlying asset and plays a crucial role in calculating the fair value of options.
Option | Underlying Asset Price | Standard Deviation |
---|---|---|
Call Option | $100 | 0.35 |
Put Option | $50 | 0.45 |
Call Option | $80 | 0.25 |
Portfolio Risk and Correlation
Correlation measures the relationship between the returns of different assets in a portfolio. By combining assets with lower correlation, investors can construct portfolios to mitigate risk and improve diversification.
Asset | Return (%) | Correlation with S&P 500 |
---|---|---|
Stock A | 3.12 | 0.57 |
Stock B | -0.45 | 0.28 |
Stock C | 2.01 | 0.82 |
Credit Scoring and Z-Scores
Z-scores are commonly used in credit scoring models to determine the creditworthiness of individuals or companies. Lenders rely on these scores to assess the probability of default and make informed lending decisions.
Borrower | Income | Z-Score |
---|---|---|
John Doe | $50,000 | 0.85 |
Jane Smith | $75,000 | 0.92 |
Mark Johnson | $100,000 | 1.16 |
Actuarial Science and Life Expectancy
In actuarial science, the standard normal distribution is used to model the life expectancy of individuals. By analyzing mortality rates and applying statistical techniques, insurance companies can assess the risk associated with different life insurance policies.
Age Group | Male | Female |
---|---|---|
30-39 | 45 | 48 |
40-49 | 35 | 40 |
50-59 | 25 | 30 |
Quality Control and Control Charts
Control charts are used in quality control to monitor and analyze process data. By assuming a normally distributed process, deviations from the mean (average) can be measured and corrective actions taken.
Sample Size | Average | Standard Deviation |
---|---|---|
25 | 3.12 | 0.42 |
30 | 2.87 | 0.54 |
20 | 3.20 | 0.39 |
Economics and Income Distribution
Standard normal distribution is often used to analyze income distribution within a population. It helps economists understand the income inequality, measure poverty rates, and design policies for wealth redistribution.
Income Group | Percentage of Population | Z-Score |
---|---|---|
Low Income | 15% | -1.25 |
Middle Income | 55% | 0.20 |
High Income | 30% | 1.45 |
Psychometrics and IQ Scores
IQ scores are often assumed to follow a standard normal distribution. They are used in psychometrics to measure intelligence and compare individuals’ cognitive abilities.
Person | IQ Score | Z-Score |
---|---|---|
John | 115 | 0.85 |
Sarah | 100 | 0 |
Robert | 130 | 1.25 |
Marketing and Customer Segmentation
In marketing, the standard normal distribution is used to segment customers based on various attributes, such as purchasing patterns, preferences, or brand loyalty. This helps marketers target specific customer groups and tailor their strategies accordingly.
Customer Segment | Average Purchase Amount | Z-Score |
---|---|---|
High Spenders | $500 | 0.80 |
Moderate Spenders | $250 | -0.20 |
Low Spenders | $100 | -1.15 |
The standard normal distribution is a fundamental concept in various fields, including finance, actuarial science, economics, and marketing. By understanding its applications, professionals and researchers can leverage its power to make informed decisions, evaluate risks, and study complex phenomena.
Frequently Asked Questions
Applications With Standard Normal Distribution
What is the standard normal distribution?
What are the applications of the standard normal distribution?
How is the standard normal distribution different from other normal distributions?
How do I standardize data using the standard normal distribution?
Can I convert any normal distribution to a standard normal distribution?
What is the significance of the standard normal table or z-table?
How can I find the probability for a specific value in the standard normal distribution?
Can the standard normal distribution be used for discrete data?
Are there any limitations or assumptions associated with the standard normal distribution?
Is it possible to transform any non-normal distribution into a standard normal distribution?